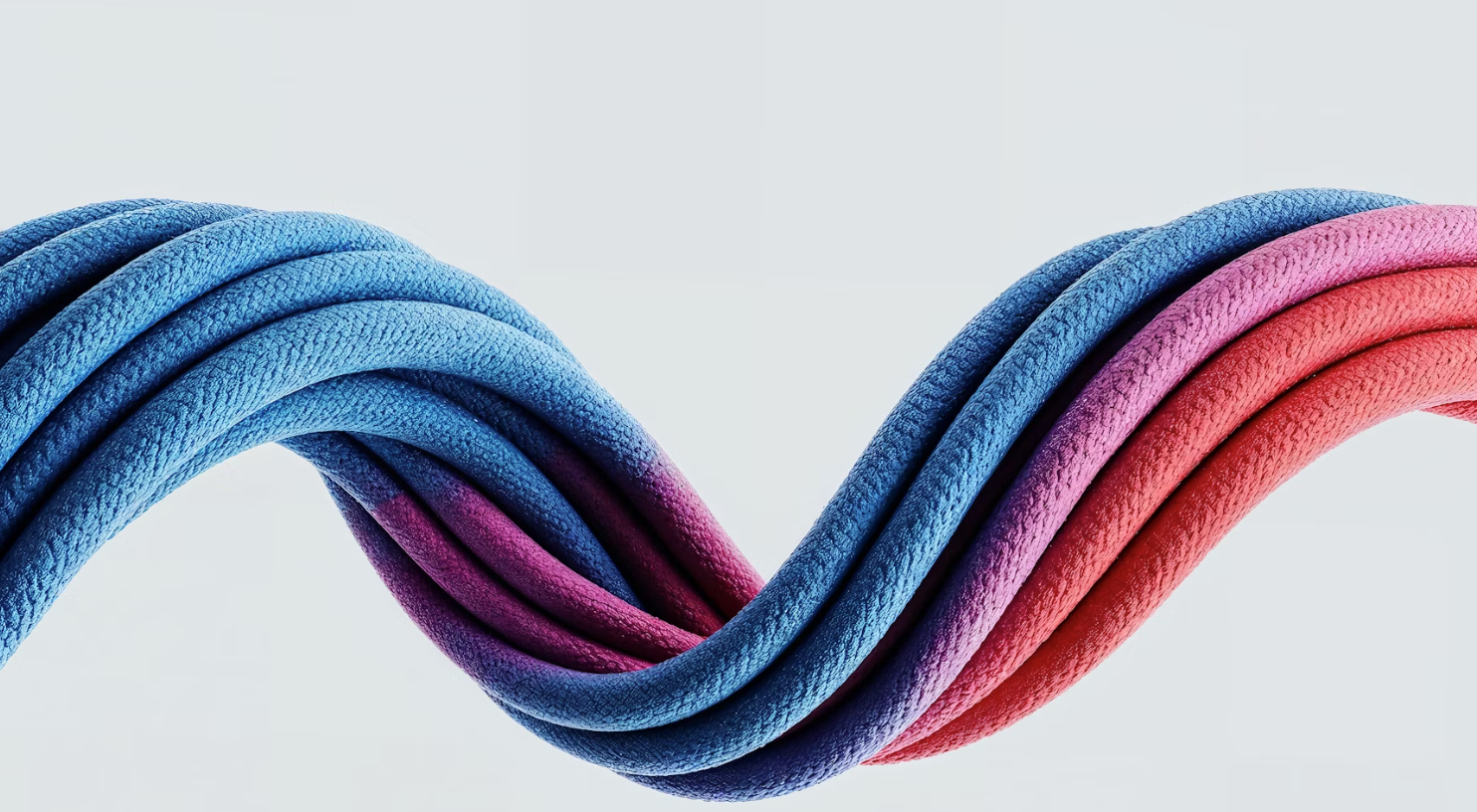
AI Frontiers 2024: Using the RDI Framework
A Research-Driven Deep Dive into Bottlenecks, Breakthroughs, and the Path Forward
-
Overview
-
Series Introduction
We introduce the series, the project undertaken and overview of the methodology.
-
Methodology
We conducted 42 in-depth, semi-structured interviews with a diverse group of AI stakeholders: primarily AI researchers, along with startup founders and industry experts.
-
Who We Interviewed
To gain a comprehensive perspective, we prioritized in-depth conversations with individuals actively involved in AI research and development. Our interviewees represented three key groups.
-
Secondary Research
The team used existing scholarly articles, conference proceedings, and white papers to get insights into the prevailing trends, persistent challenges, and burgeoning opportunities.
-
Scope and Limitations
We provide a description of who we talked to and what we explored in the conversations. This provides context for the subsequent findings.
-
-
Findings
-
5 Emerging Trends in AI
We examine trends from primary and secondary sources in the evolving field of Generative AI
-
6 Drivers of AI Innovation
Here we provide a breakdown of recent AI breakthroughs, new datasets, and emerging techniques, drawing from the primary and secondary research.
-
7 Bottlenecks in AI
We share high-level bottlenecks for AI research, startups, and practitioners.
-
3 Enablers and 5 Barriers to AI Research Breakthroughs within Large Technology Organizations
This article is geared towards larger tech companies.
-
-
Deep Dives
-
AI Data Centers & Compute: Challenges & Opportunities
We explore the major trends, pain points, challenges and opportunities for one of the major bottlenecks of AI.
-
Data Challenges and Trends for Foundation Models
We explore various pain points and difficulties encountered in data acquisition, processing, and training.
-
Novel and Evolving Techniques in AI
We provide a review of novel and evolving methods used by AI researchers and engineers.
-
How AI Researchers Are Evaluating Models
Beyond benchmarks, researchers are exploring creative and advanced ways to evaluate their models.
-
The Real Bottleneck in GenAI Adoption – Skills and Knowledge Gaps, Not Just UX
We explore 2 major bottlenecks to AI Adoption
-
-
Discussions
-
Are AI Benchmarks Obsolete? The Rise of User-Centric Evaluation
We dive into benchmarks and competitions for AI researchers
-
Is Compute Really a Bottleneck for AI? Inside AI Data Centers
We examine one of the most cited bottlenecks in AI by researchers and startups alike.
-
Investing in AI Education: A Strategic Imperative
The integration of Artificial Intelligence (AI) is no longer a futuristic aspiration but a present-day necessity for businesses striving for competitive advantage, enhanced productivity, and innovative growth. Senior leadership teams face mounting pressure to not only adopt AI but to do so effectively, mitigating risks and realizing tangible value.
-
-
Conclusion